Author: Jason Brownlee
The weights of artificial neural networks must be initialized to small random numbers.
This is because this is an expectation of the stochastic optimization algorithm used to train the model, called stochastic gradient descent.
To understand this approach to problem solving, you must first understand the role of nondeterministic and randomized algorithms as well as the need for stochastic optimization algorithms to harness randomness in their search process.
In this post, you will discover the full background as to why neural network weights must be randomly initialized.
After reading this post, you will know:
- About the need for nondeterministic and randomized algorithms for challenging problems.
- The use of randomness during initialization and search in stochastic optimization algorithms.
- That stochastic gradient descent is a stochastic optimization algorithm and requires the random initialization of network weights.
Let’s get started.
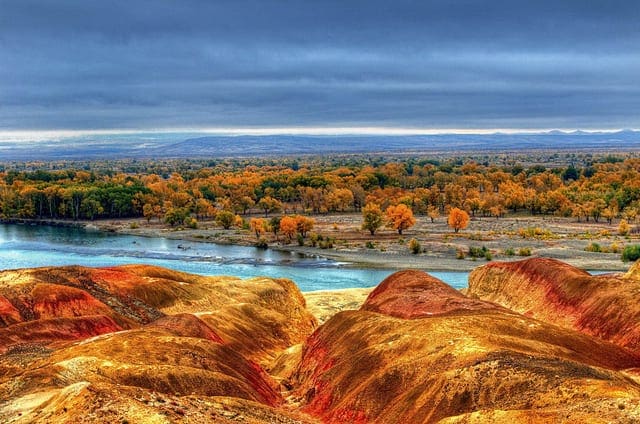
Why Initialize a Neural Network with Random Weights?
Photo by lwtt93, some rights reserved.
Overview
This post is divided into 4 parts; they are:
- Deterministic and Non-Deterministic Algorithms
- Stochastic Search Algorithms
- Random Initialization in Neural Networks
- Initialization Methods
Deterministic and Non-Deterministic Algorithms
Classical algorithms are deterministic.
An example is an algorithm to sort a list.
Given an unsorted list, the sorting algorithm, say bubble sort or quick sort, will systematically sort the list until you have an ordered result. Deterministic means that each time the algorithm is given the same list, it will execute in exactly the same way. It will make the same moves at each step of the procedure.
Deterministic algorithms are great as they can make guarantees about best, worst, and average running time. The problem is, they are not suitable for all problems.
Some problems are hard for computers. Perhaps because of the number of combinations; perhaps because of the size of data. They are so hard because a deterministic algorithm cannot be used to solve them efficiently. The algorithm may run, but will continue running until the heat death of the universe.
An alternate solution is to use nondeterministic algorithms. These are algorithms that use elements of randomness when making decisions during the execution of the algorithm. This means that a different order of steps will be followed when the same algorithm is rerun on the same data.
They can rapidly speed up the process of getting a solution, but the solution will be approximate, or “good,” but often not the “best.” Nondeterministic algorithms often cannot make strong guarantees about running time or the quality of the solution found.
This is often fine as the problems are so hard that any good solution will often be satisfactory.
Stochastic Search Algorithms
Search problems are often very challenging and require the use of nondeterministic algorithms that make heavy use of randomness.
The algorithms are not random per se; instead they make careful use of randomness. They are random within a bound and are referred to as stochastic algorithms.
The incremental, or step-wise, nature of the search often means the process and the algorithms are referred to as an optimization from an initial state or position to a final state or position. For example, stochastic optimization problem or a stochastic optimization algorithm.
Some examples include the genetic algorithm, simulated annealing, and stochastic gradient descent.
The search process is incremental from a starting point in the space of possible solutions toward some good enough solution.
They share common features in their use of randomness, such as:
- Use of randomness during initialization.
- Use of randomness during the progression of the search.
We know nothing about the structure of the search space. Therefore, to remove bias from the search process, we start from a randomly chosen position.
As the search process unfolds, there is a risk that we are stuck in an unfavorable area of the search space. Using randomness during the search process gives some likelihood of getting unstuck and finding a better final candidate solution.
The idea of getting stuck and returning a less-good solution is referred to as getting stuck in a local optima.
These two elements of random initialization and randomness during the search work together.
They work together better if we consider any solution found by the search as provisional, or a candidate, and that the search process can be performed multiple times.
This gives the stochastic search process multiple opportunities to start and traverse the space of candidate solutions in search of a better candidate solution–a so-called global optima.
The navigation of the space of candidate solutions is often described using the analogy of a one- or two-landscape of mountains and valleys (e.g. like a fitness landscape). If we are maximizing a score during the search, we can think of small hills in the landscape as a local optima and the largest hills as the global optima.
This is a fascinating area of research, an area where I have some background. For example, see my book:
Random Initialization in Neural Networks
Artificial neural networks are trained using a stochastic optimization algorithm called stochastic gradient descent.
The algorithm uses randomness in order to find a good enough set of weights for the specific mapping function from inputs to outputs in your data that is being learned. It means that your specific network on your specific training data will fit a different network with a different model skill each time the training algorithm is run.
This is a feature, not a bug.
I write about this issue more in the post:
As described in the previous section, stochastic optimization algorithms such as stochastic gradient descent use randomness in selecting a starting point for the search and in the progression of the search.
Specifically, stochastic gradient descent requires that the weights of the network are initialized to small random values (random, but close to zero, such as in [0.0, 0.1]). Randomness is also used during the search process in the shuffling of the training dataset prior to each epoch, which in turn results in differences in the gradient estimate for each batch.
You can learn more about stochastic gradient descent in this post:
The progression of the search or learning of a neural network is referred to as convergence. The discovering of a sub-optimal solution or local optima is referred to as premature convergence.
Training algorithms for deep learning models are usually iterative in nature and thus require the user to specify some initial point from which to begin the iterations. Moreover, training deep models is a sufficiently difficult task that most algorithms are strongly affected by the choice of initialization.
— Page 301, Deep Learning, 2016.
The most effective way to evaluate the skill of a neural network configuration is to repeat the search process multiple times and report the average performance of the model over those repeats. This gives the configuration the best chance to search the space from multiple different sets of initial conditions. Sometimes this is called a multiple restart or multiple-restart search.
You can learn more about the effective evaluation of neural networks in this post:
Why Not Set Weights to Zero?
We can use the same set of weights each time we train the network; for example, you could use the values of 0.0 for all weights.
In this case, the equations of the learning algorithm would fail to make any changes to the network weights, and the model will be stuck. It is important to note that the bias weight in each neuron is set to zero by default, not a small random value.
Specifically, nodes that are side-by-side in a hidden layer connected to the same inputs must have different weights for the learning algorithm to update the weights.
This is often referred to as the need to break symmetry during training.
Perhaps the only property known with complete certainty is that the initial parameters need to “break symmetry” between different units. If two hidden units with the same activation function are connected to the same inputs, then these units must have different initial parameters. If they have the same initial parameters, then a deterministic learning algorithm applied to a deterministic cost and model will constantly update both of these units in the same way.
— Page 301, Deep Learning, 2016.
When to Initialize to the Same Weights?
We could use the same set of random numbers each time the network is trained.
This would not be helpful when evaluating network configurations.
It may be helpful in order to train the same final set of network weights given a training dataset in the case where a model is being used in a production environment.
You can learn more about fixing the random seed for neural networks developed with Keras in this post:
Initialization Methods
Traditionally, the weights of a neural network were set to small random numbers.
The initialization of the weights of neural networks is a whole field of study as the careful initialization of the network can speed up the learning process.
Modern deep learning libraries, such as Keras, offer a host of network initialization methods, all are variations of initializing the weights with small random numbers.
For example, the current methods are available in Keras at the time of writing for all network types:
- Zeros: Initializer that generates tensors initialized to 0.
- Ones: Initializer that generates tensors initialized to 1.
- Constant: Initializer that generates tensors initialized to a constant value.
- RandomNormal: Initializer that generates tensors with a normal distribution.
- RandomUniform: Initializer that generates tensors with a uniform distribution.
- TruncatedNormal: Initializer that generates a truncated normal distribution.
- VarianceScaling: Initializer capable of adapting its scale to the shape of weights.
- Orthogonal: Initializer that generates a random orthogonal matrix.
- Identity: Initializer that generates the identity matrix.
- lecun_uniform: LeCun uniform initializer.
- glorot_normal: Glorot normal initializer, also called Xavier normal initializer.
- glorot_uniform: Glorot uniform initializer, also called Xavier uniform initializer.
- he_normal: He normal initializer.
- lecun_normal: LeCun normal initializer.
- he_uniform: He uniform variance scaling initializer.
See the documentation for more details.
Out of interest, the default initializers chosen by Keras developers for different layer types are as follows:
- Dense (e.g. MLP): glorot_uniform
- LSTM: glorot_uniform
- CNN: glorot_uniform
You can learn more about “glorot_uniform“, also called “Xavier normal“, named for the developer of the method Xavier Glorot, in the paper:
There is no single best way to initialize the weights of a neural network.
Modern initialization strategies are simple and heuristic. Designing improved initialization strategies is a difficult task because neural network optimization is not yet well understood. […] Our understanding of how the initial point affects generalization is especially primitive, offering little to no guidance for how to select the initial point.
— Page 301, Deep Learning, 2016.
It is one more hyperparameter for you to explore and test and experiment with on your specific predictive modeling problem.
Do you have a favorite method for weight initialization?
Let me know in the comments below.
Further Reading
This section provides more resources on the topic if you are looking to go deeper.
Books
- Deep Learning, 2016.
Articles
- Nondeterministic algorithm on Wikipedia
- Randomized algorithm on Wikipedia
- Stochastic optimization on Wikipedia
- Stochastic gradient descent on Wikipedia
- Fitness landscape on Wikipedia
- Neural Network FAQ
- Keras Weight Initialization
- Understanding the difficulty of training deep feedforward neural networks, 2010.
Discussion
- What are good initial weights in a neural network?
- Why should weights of Neural Networks be initialized to random numbers?
- What are good initial weights in a neural network?
Summary
In this post, you discovered why neural network weights must be randomly initialized.
Specifically, you learned:
- About the need for nondeterministic and randomized algorithms for challenging problems.
- The use of randomness during initialization and search in stochastic optimization algorithms.
- That stochastic gradient descent is a stochastic optimization algorithm and requires the random initialization of network weights.
Do you have any questions?
Ask your questions in the comments below and I will do my best to answer.
The post Why Initialize a Neural Network with Random Weights? appeared first on Machine Learning Mastery.