Author: Jason Brownlee
A final machine learning model is one trained on all available data and is then used to make predictions on new data.
A problem with most final models is that they suffer variance in their predictions.
This means that each time you fit a model, you get a slightly different set of parameters that in turn will make slightly different predictions. Sometimes more and sometimes less skillful than what you expected.
This can be frustrating, especially when you are looking to deploy a model into an operational environment.
In this post, you will discover how to think about model variance in a final model and techniques that you can use to reduce the variance in predictions from a final model.
After reading this post, you will know:
- The problem with variance in the predictions made by a final model.
- How to measure model variance and how variance is addressed generally when estimating parameters.
- Techniques you can use to reduce the variance in predictions made by a final model.
Let’s get started.
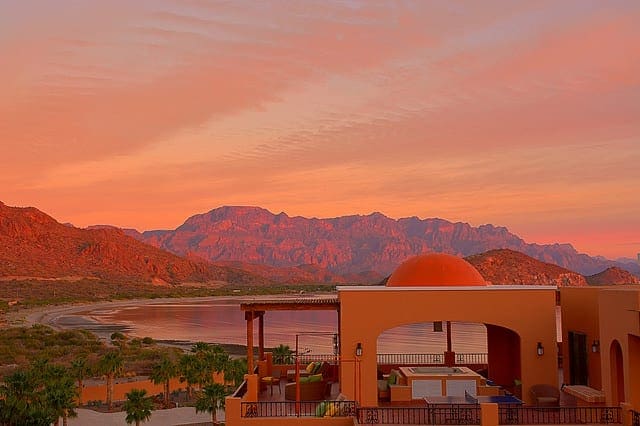
How to Reduce Variance in a Final Machine Learning Model
Photo by Kirt Edblom, some rights reserved.
Final Model
Once you have discovered which model and model hyperparameters result in the best skill on your dataset, you’re ready to prepare a final model.
A final model is trained on all available data, e.g. the training and the test sets.
It is the model that you will use to make predictions on new data were you do not know the outcome.
The final model is the outcome of your applied machine learning project.
To learn more about preparing a final model, see the post:
Bias and Variance
The bias-variance trade-off is a conceptual idea in applied machine learning to help understand the sources of error in models.
- Bias refers to assumptions in the learning algorithm that narrow the scope of what can be learned. This is useful as it can accelerate learning and lead to stable results, at the cost of the assumption differing from reality.
- Variance refers to the sensitivity of the learning algorithm to the specifics of the training data, e.g. the noise and specific observations. This is good as the model will be specialized to the data at the cost of learning random noise and varying each time it is trained on different data.
The bias-variance tradeoff is a conceptual tool to think about these sources of error and how they are always kept in balance.
More bias in an algorithm means that there is less variance, and the reverse is also true.
You can learn more about the bias-variance tradeoff in this post:
You can control this balance.
Many machine learning algorithms have hyperparameters that directly or indirectly allow you to control the bias-variance tradeoff.
For example, the k in k-nearest neighbors is one example. A small k results in predictions with high variance and low bias. A large k results in predictions with a small variance and a large bias.
The Problem of Variance in Final Models
Most final models have a problem: they suffer from variance.
Each time a model is trained by an algorithm with high variance, you will get a slightly different result.
The slightly different model in turn will make slightly different predictions, for better or worse.
This is a problem with training a final model as we are required to use the model to make predictions on real data where we do not know the answer and we want those predictions to as good as possible.
We want to the best possible version of the model that we can get.
We want the variance to play out in our favor.
If we can’t achieve that, at least we want the variance to not fall against us when making predictions.
Measure Variance in the Final Model
There are two common sources of variance in a final model:
- The noise in the training data.
- The use of randomness in the machine learning algorithm.
The first type we introduced above.
The second type impacts those algorithms that harness randomness during learning.
Three common examples include:
- Choice of random split points in random forest.
- Random weight initialization in neural networks.
- Shuffling training data in stochastic gradient descent.
You can measure both types of variance in your specific model using your training data.
- Measure Algorithm Variance: The variance introduced by the stochastic nature of the algorithm can be measured by repeating the evaluation of the algorithm on the same training dataset and calculating the variance or standard deviation of the model skill.
- Measure Training Data Variance: The variance introduced by the training data can be measured by repeating the evaluation of the algorithm on different samples of training data, but keeping the seed for the pseudorandom number generator fixed then calculating the variance or standard deviation of the model skill.
Often, the combined variance is estimated by running repeated k-fold cross-validation on a training dataset then calculating the variance or standard deviation of the model skill.
Reduce Variance of an Estimate
If we want to reduce the amount of variance in a prediction, we must add bias.
Consider the case of a simple statistical estimate of a population parameter, such as estimating the mean from a small random sample of data.
A single estimate of the mean will have high variance and low bias.
This is intuitive because if we repeated this process 30 times and calculated the standard deviation of the estimated mean values, we would see a large spread.
The solutions for reducing the variance are also intuitive.
Repeat the estimate on many different small samples of data from the domain and calculate the mean of the estimates, leaning on the central limit theorem.
The mean of the estimated means will have a lower variance. We have increased the bias by assuming that the average of the estimates will be a more accurate estimate than a single estimate.
Another approach would be to dramatically increase the size of the data sample on which we estimate the population mean, leaning on the law of large numbers.
Reduce Variance of a Final Model
The principles used to reduce the variance for a population statistic can also be used to reduce the variance of a final model.
We must add bias.
Depending on the specific form of the final model (e.g. tree, weights, etc.) you can get creative with this idea.
Below are three approaches that you may want to try.
If possible, I recommend designing a test harness to experiment and discover an approach that works best or makes the most sense for your specific data set and machine learning algorithm.
1. Ensemble Predictions from Final Models
Instead of fitting a single final model, you can fit multiple final models.
Together, the group of final models may be used as an ensemble.
For a given input, each model in the ensemble makes a prediction and the final output prediction is taken as the average of the predictions of the models.
A sensitivity analysis can be used to measure the impact of ensemble size on prediction variance.
2. Ensemble Parameters from Final Models
As above, multiple final models can be created instead of a single final model.
Instead of calculating the mean of the predictions from the final models, a single final model can be constructed as an ensemble of the parameters of the group of final models.
This would only make sense in cases where each model has the same number of parameters, such as neural network weights or regression coefficients.
For example, consider a linear regression model with three coefficients [b0, b1, b2]. We could fit a group of linear regression models and calculate a final b0 as the average of b0 parameters in each model, and repeat this process for b1 and b2.
Again, a sensitivity analysis can be used to measure the impact of ensemble size on prediction variance.
3. Increase Training Dataset Size
Leaning on the law of large numbers, perhaps the simplest approach to reduce the model variance is to fit the model on more training data.
In those cases where more data is not readily available, perhaps data augmentation methods can be used instead.
A sensitivity analysis of training dataset size to prediction variance is recommended to find the point of diminishing returns.
Fragile Thinking
There are approaches to preparing a final model that aim to get the variance in the final model to work for you rather than against you.
The commonality in these approaches is that they seek a single best final model.
Two examples include:
- Why not fix the random seed? You could fix the random seed when fitting the final model. This will constrain the variance introduced by the stochastic nature of the algorithm.
- Why not use early stopping? You could check the skill of the model against a holdout set during training and stop training when the skill of the model on the hold set starts to degrade.
I would argue that these approaches and others like them are fragile.
Perhaps you can gamble and aim for the variance to play-out in your favor. This might be a good approach for machine learning competitions where there is no real downside to losing the gamble.
I won’t.
I think it’s safer to limit the aim for the best average performance and limit the downside.
I think that the trick with navigating the bias-variance tradeoff for a final model is to think in samples, not in terms of single models. To optimize for average model performance.
Further Reading
This section provides more resources on the topic if you are looking to go deeper.
- How to Train a Final Machine Learning Model
- Gentle Introduction to the Bias-Variance Trade-Off in Machine Learning
- Bias-Variance Tradeoff on Wikipedia
- Checkpoint Ensembles: Ensemble Methods from a Single Training Process, 2017.
Summary
In this post, you discovered how to think about model variance in a final model and techniques that you can use to reduce the variance in predictions from a final model.
Specifically, you learned:
- The problem with variance in the predictions made by a final model.
- How to measure model variance and how variance is addressed generally when estimating parameters.
- Techniques you can use to reduce the variance in predictions made by a final model.
Do you have any questions?
Ask your questions in the comments below and I will do my best to answer.
The post How to Reduce Variance in a Final Machine Learning Model appeared first on Machine Learning Mastery.