Author:
There’s nothing quite like driving through Los Angeles on a perfectly sunny day. But for drivers, the beauty of Southern California’s great weather and scenery is ruined by one thing: traffic.
According to a report by INRIX, my hometown is the worst city in the world for traffic, with a record of 102 hours of congestion during peak hours in 2017. My classmate, Ericson Hernandez, comes from New York City, which is ranked third globally for its traffic woes. Together, we decided to use machine learning to figure out the roots of bad traffic, including elements like road damage from potholes and cracks, and make rides around our beautiful cities enjoyable again.
As Ericson and I started studying electrical engineering at Loyola Marymount University, we began to develop an interest in a relatively new topic to the engineering world: machine learning. Our professor, Dr. Lei Huang, encouraged us to pick a project that we were passionate about, and Ericson and I wanted to use technology to tackle problems in the real world—such as helping the communities around us with road development.
This summer, we looked at previous research projects on detecting road cracks, and pondered how we could improve the algorithm and apply it to Los Angeles communities. We decided to use TensorFlow, Google’s open-source machine learning platform, to train a model that could quickly identify potholes and dangerous road cracks from camera footage of L.A. roads.
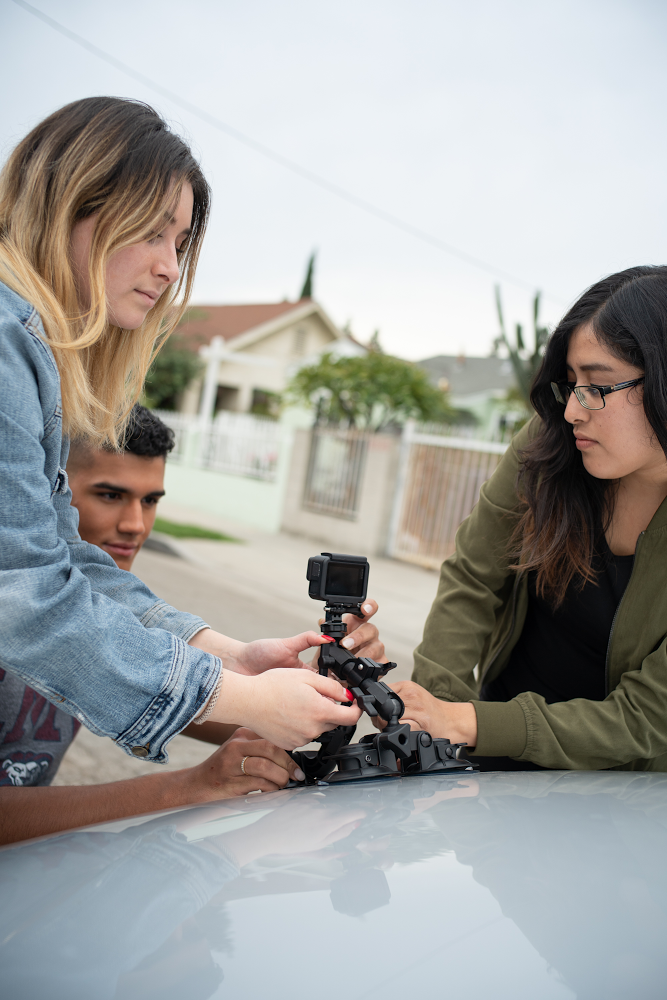
Students mount their camera before heading out to collect data.
Construction companies and cities could use this technology to identify which roads need fixing the most. With safer driving conditions and efficient road-work repairs, traffic in major cities could dramatically decrease, allowing for people to travel in a quick, safe and enjoyable manner.
And that way, driving through Los Angeles can be about enjoying the view, not grumbling at the traffic.